The 8-Minute Rule for "Overcoming Challenges in Implementing and Maintaining an AI Stack"
Getting over Problem in Implementing and Preserving an AI Stack
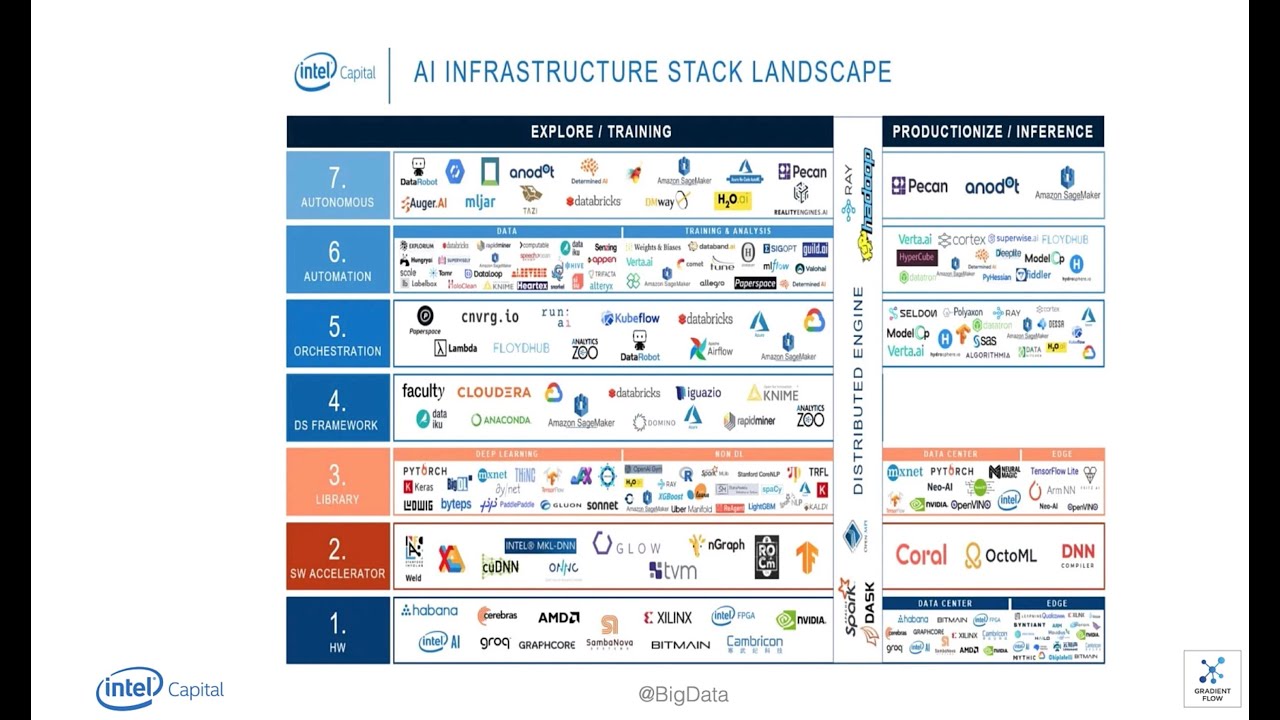
Implementing and keeping an AI bundle may be a tough venture for any company. While the perks of leveraging man-made knowledge (AI) innovations are well-known, there are actually several barriers to gotten rid of when it happens to successfully carrying out and preserving an AI stack. In this post, we will look into the vital difficulty dealt with through associations and discuss methods to gotten over them.
1. Data Quality and Availability:
One of the main problem in applying an AI bundle is making certain the high quality and accessibility of information. AI algorithms greatly depend on high-quality, diverse, and clean datasets for accurate predictions and knowledge. Having said that, organizations commonly have a hard time along with information silos, unfinished or wrong record, or not enough information amount. To deal with this obstacle, institutions should invest in sturdy data administration strategies that guarantee information is properly managed, standardized, legitimized, and available around various units.
2. Talent Gap:
Another notable problem is the scarcity of skillful professionals who can develop, create, carry out, and maintain AI devices. The demand for AI skill much goes beyond the supply offered in the task market today. To bridge this gap, associations need to focus on drawing in leading skill via very competitive settlement deals and offering recurring training opportunities to upskill existing workers.
3. Honest Considerations:
AI raises vital honest factors that need to be attended to throughout application. For example, prejudices existing in training datasets may lead to unethical outcomes or prejudiced methods when deployed in real-world cases. Associations must guarantee transparency in their formulas' decision-making methods while proactively observing for predispositions throughout the advancement lifecycle.
4. Commercial infrastructure Requirements:
Implementing an reliable AI heap requires a sturdy IT commercial infrastructure qualified of dealing with large volumes of data processing power-intensive activities efficiently. Associations may encounter difficulty related to scalability problems or out-of-date components commercial infrastructure that limits successful deployment of AI designs at scale. Spending in modern-day hardware facilities such as high-performance processing sets or cloud-based options can easily help eliminated these problem.
5. Another Point of View with Existing Systems:
Institutions commonly struggle with integrating AI systems flawlessly into their existing modern technology landscape. Legacy bodies and out-of-date software application architectures may pose significant difficulty when trying to integrate AI abilities. A careful examination of existing systems, identity of prospective assimilation factors, and cooperation between various crews are crucial to make certain a hassle-free combination process.
6. Safety and security and Privacy Concerns:
AI systems take care of substantial volumes of sensitive record, producing security and privacy worries a extremely important obstacle. Organizations need to have to execute strong safety and security procedure to shield information from unwarranted gain access to or breaches. Additionally, conformity along with record protection regulations such as the General Data Protection Regulation (GDPR) is critical to steer clear of legal implications.
7. Continuous Monitoring and Maintenance:
Keeping an AI heap demands continuous screen, upkeep, and improve over opportunity. Styles require frequent retraining or fine-tuning to guarantee they continue to be exact and relevant in developing service environments. Institutions should set up very clear methods for keeping an eye on style efficiency, recognizing abnormalities or drifts, and applying important updates or improvements.
8. Come back on Investment (ROI):
Implementing an AI bundle includes considerable expenditure in phrases of sources, opportunity, and finances. To validate this expenditure, associations require to evaluate the possible yield on investment accurately. This demands establishing clear objectives from the beginning, describing key efficiency signs (KPIs), and frequently assessing the effect of AI executions against these metrics.
In final thought, carrying out and keeping an AI stack shows many challenges that companies should eliminated for productive adoption. By dealing with concerns related to information premium and supply, skill void, reliable factors, framework criteria, combination difficulties protection problems continuous screen servicing as properly as ROI evaluation companies can easily navigate these challenges effectively making sure productive application maintenance of their AI heap.